Boosting Learning with Incomplete Data
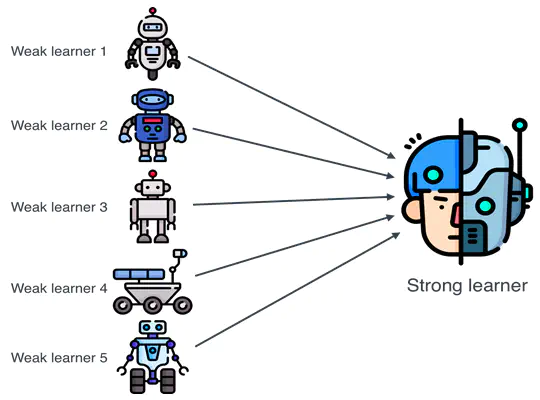
Boosting has emerged as one of the most powerful machine learning techniques over the past three decades, capturing the attention of numerous researchers. The majority of advancements in this field, however, have primarily focused on numerical implementation procedures tailored for handling datasets with complete observations, often lacking theoretical justifications. In these projects, we explore different strategies to accomondate response variable subject to missingness or intervel censoring. We implement the proposed methods using a functional gradient descent algorithm and rigorously establish their theoretical properties, including optimization convergence and consistency of the proposed estimators. Numerical studies demonstrate that the proposed methods exhibit satisfactory performance in finite sample settings.